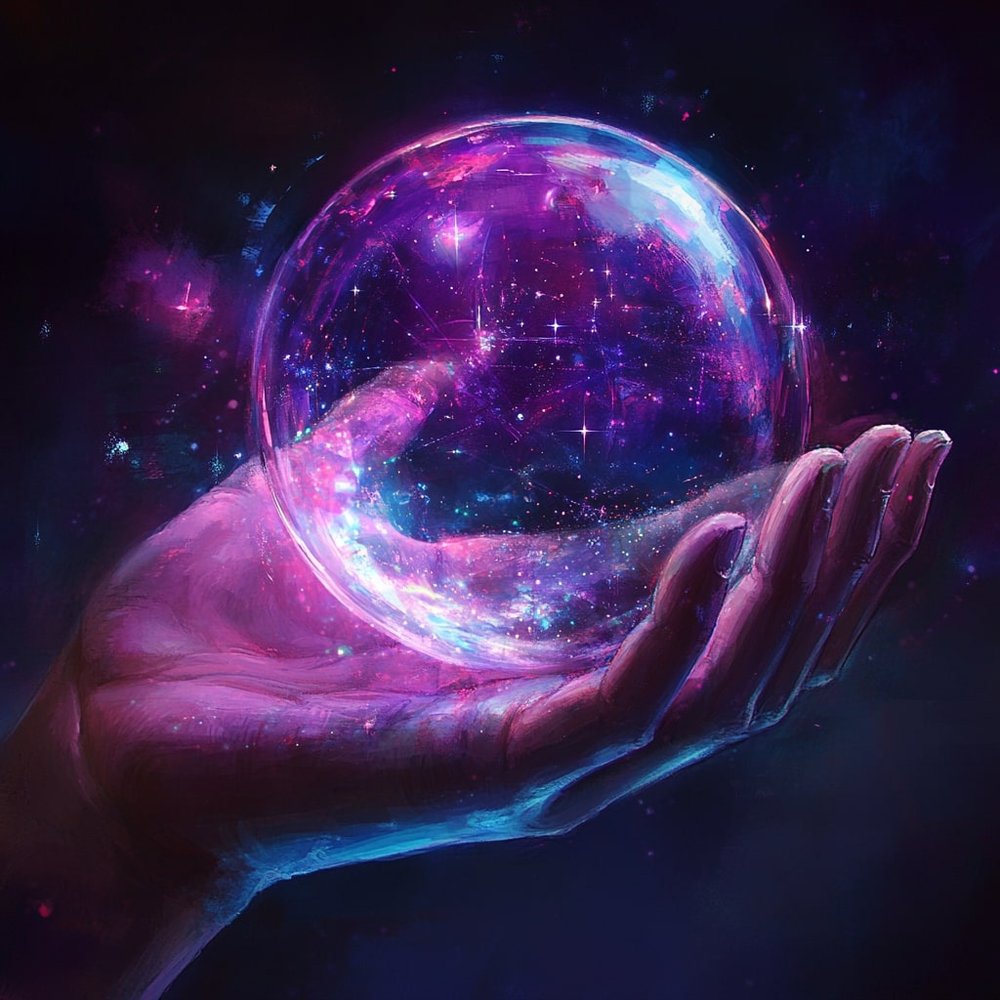
Predictive analytics is a type of data analysis that uses historical data to make predictions about future trends. businesses use predictive analytics to make decisions about everything from pricing and inventory management to marketing and customer service. In order to be effective, predictive analytics must be based on a large and diverse dataset. The more data points there are, the more accurate the predictions will be. businesses that have access to large datasets, such as customer purchase history or social media interactions, have a significant advantage when it comes to using predictive analytics. By understanding past patterns, they can make better decisions about the future. As predictive analytics becomes more widely used, businesses that don't embrace it will find themselves at a competitive disadvantage.
Predictive analytics involves using statistical algorithms, machine learning techniques, and data mining to analyze historical data and predict future outcomes. It is widely applied across various business functions to enhance decision-making. For example, in marketing, predictive analytics can identify customer behaviors and preferences, enabling targeted campaigns that improve conversion rates. In finance, it can assess credit risk and detect fraudulent activities. Retailers use it for demand forecasting to optimize inventory levels and reduce waste.
The effectiveness of predictive analytics relies on data quality, volume, and diversity. Businesses that integrate data from multiple sources—like customer transactions, social media, and web analytics—can create more accurate models. However, data privacy and ethical considerations must be managed carefully to maintain customer trust.
The process typically involves several steps:
-
Data Collection and Preparation: Gathering and cleaning data from various sources, such as CRM systems, transaction logs, or external datasets.
-
Model Building: Selecting and training algorithms to identify patterns and trends within the data.
-
Model Evaluation and Validation: Testing the model’s performance against unseen data to ensure accuracy and reliability.
-
Deployment and Monitoring: Implementing the model into the business workflow and continuously monitoring its performance to refine and improve predictions.
With advancements in AI and machine learning, predictive analytics is becoming more accessible and powerful, allowing even small businesses to leverage insights for growth and efficiency. Companies that fail to adopt these data-driven approaches risk falling behind competitors who capitalize on the strategic advantages predictive analytics can provide.
Looking ahead, the scope of predictive analytics is expected to expand further, integrating real-time data for even more dynamic and responsive decision-making processes. As this field grows, businesses must invest in the right tools, talent, and data governance practices to maximize its potential benefits while mitigating risks related to data security and privacy.